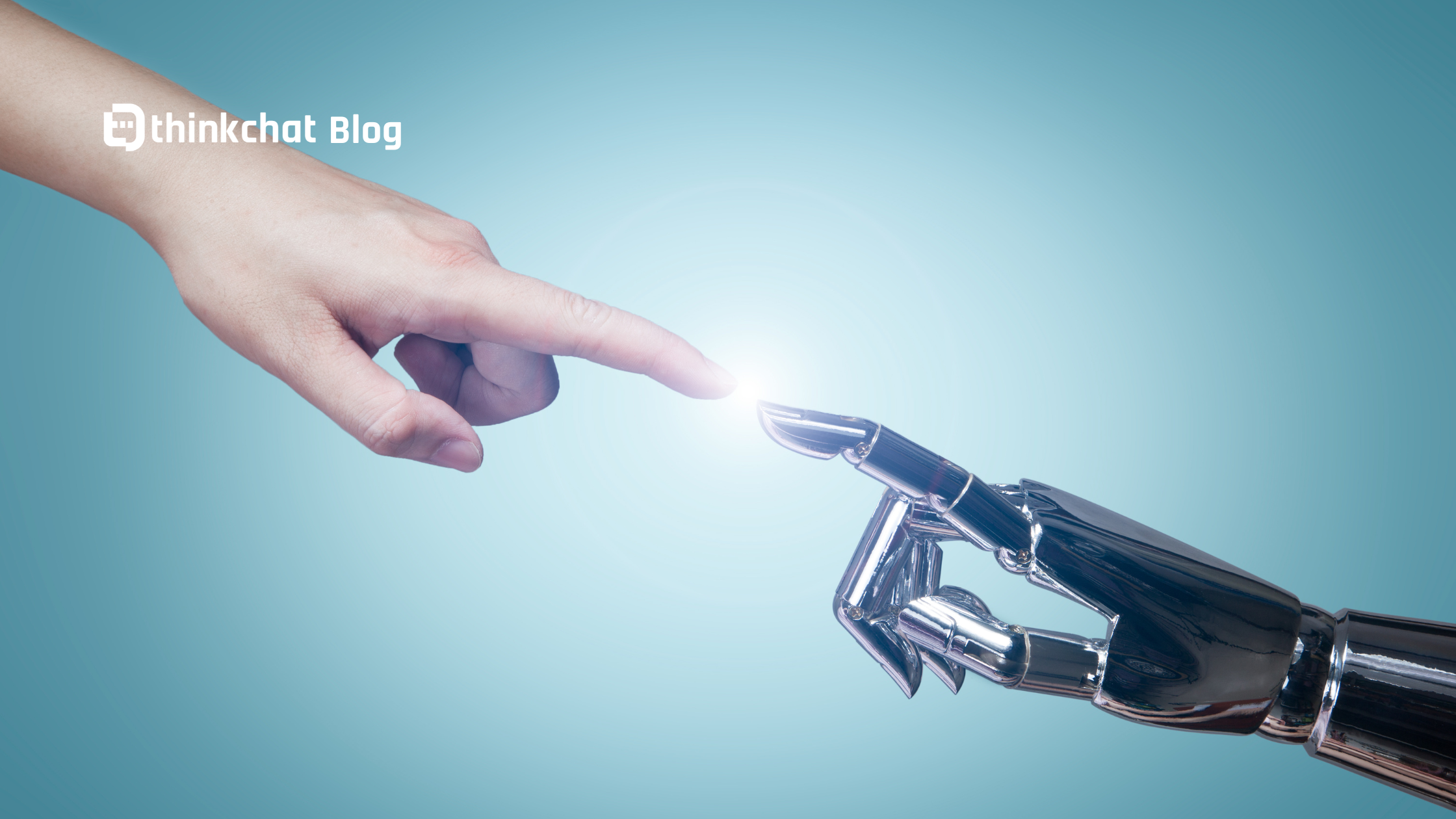
Admin
How Close is AI to Human-Level Intelligence?
Advanced language models, such as OpenAIs o1, have reignited the debate about the possibility of achieving artificial general intelligence (AGI). However, many experts argue that these models alone will not reach this milestone.
In September, OpenAI released its latest AI system with an ambitious promise: presenting o1, a suite of advanced language models, as an AI closer to how a person thinks compared to previous versions. OpenAI, based in San Francisco, claims that o1 represents a new level of AI capability.
This release has reignited a debate that has existed for decades: how much longer until a machine can perform all the cognitive tasks that human brains can handle, such as generalizing from one task to another, abstract reasoning, planning, and choosing which aspects of the world to investigate and learn from? Such an artificial general intelligence (AGI) could tackle complex problems like climate change, pandemics, or cures for diseases like cancer and Alzheimers. However, such enormous power would also bring uncertainty and risks to humanity. As Yoshua Bengio, a deep-learning researcher at the University of Montreal, warns, “bad things could happen due to the misuse of AI or by losing control of it.”
The revolution in language models in recent years has led to speculation that AGI might be tantalizingly close. However, due to how language models are built and trained, some researchers believe they will not be sufficient to achieve AGI on their own. “There are still some pieces missing,” says Bengio.
Why the AGI Debate Has Changed
The term artificial general intelligence became popular around 2007, when it was mentioned in a book edited by researchers Ben Goertzel and Cassio Pennachin. Although its precise meaning remains unclear, it generally refers to an AI system with human-like reasoning and generalization abilities. Throughout the history of AI, it has been clear that we have not yet reached AGI. For example, AlphaGo, the AI program from Google DeepMind, defeated the best human players at the board game Go, but its capabilities are limited to that one task.
The new capabilities of language models have radically changed this scenario. Like the human brain, language models possess a wide range of abilities that have led some researchers to seriously consider that some form of AGI might be imminent, or even already here.
The Training Process of Language Models
Language models like o1, Claude (from Anthropic), and Gemini (from Google) are neural networks, machine learning models loosely inspired by the brain. These networks consist of artificial neurons arranged in layers, with adjustable parameters that determine the strength of connections between them. During training, the models are fed text fragments and code. Through a process called next-token prediction, the model must predict the missing token in a sequence of text. This process allows the model to acquire the statistical structure of the language and the knowledge contained in the data.
The transformer neural network architecture has allowed language models to advance beyond previous achievements. This architecture enables a model to learn which tokens have a particularly strong influence on others, even if they are widely separated in a text sequence. This approach has been highly successful in tasks such as generating computer programs, summarizing academic papers, and answering math questions.
The Limitations of Language Models
Despite the impressive capabilities of language models, they still have limitations. For example, in tasks that require planning, o1 performs well on tasks with up to 16 steps, but its performance drops rapidly when the number of steps exceeds 20 or 40. Additionally, the model struggles with abstract reasoning and generalization, as shown in a test designed to measure progress toward AGI. These models, according to experts, cannot truly adapt to novelty because they lack the ability to recombine what they have learned to handle new tasks.
Can Language Models Achieve AGI?
The transformers that underpin language models can process and find statistical patterns not only in text but also in images and audio. While some researchers believe AGI is still out of reach, language models based on transformers already exhibit some AGI-like behaviors.
However, there are signs that transformer-based language models have limits. The data used to train these models is running out, and some researchers point out that the advances in these models are slowing down. Additionally, architectures based solely on transformers, according to Raia Hadsell from Google DeepMind, may not be enough to achieve AGI. Models that generate solutions all at once or in large chunks may bring us closer to AGI.
Building a World Model
Advances toward AGI may be inspired by ideas from neuroscientists, who argue that our intelligence results from the brains ability to build a "world model." This model allows us to imagine different courses of action, predict their consequences, plan, and reason. It also allows us to generalize skills learned in one domain to new tasks by simulating different scenarios.
In conclusion, while language models are advancing rapidly, we are still far from achieving AGI. Although these models show impressive behaviors, they are still limited in their ability to reason abstractly, adapt to new situations, and recombine knowledge to solve complex tasks. The path to AGI will be long and will require overcoming several fundamental obstacles.
Source: Nature, 2024. www.nature.com